ChatGPT is overrated because language and thinking are not the same thing
1. Language is so hot right now
As a speech pathologist, it’s great to see language in the headlines. Almost daily, I read reports of scientists, teachers, doctors, lawyers, accountants, other professionals, entrepreneurs, and students busily experimenting with language in the context of exciting artificial intelligence tools, like Open AI’s ChatGPT, and other so-called Large Language Models (LLMs).
As a lawyer and speech pathologist, I’ll admit: I’ve been a bit obsessed with LLMs for the last six months. For the first time, we have computer systems that can speak back to us fluently in something resembling our own language.
But my interest in the current generation of AI tools is waning.
Yes, LLMs produce grammatically correct and convincing answers to all sorts of questions and instructions. But they also regularly produce inaccurate “hallucinations”, with made-up facts and inferences (e.g. Dziri et al., 2022), safety lapses (e.g. Edwards, 2023), and sometimes nonsensical responses to basic questions (e.g. Bender et al., 2021). As MIT researcher Lionel Wong and colleagues recently observed in a terrific and thought-provoking paper:
“While LLMs may reasonably appear to condition on input language and answer queries under some circumstances, it is precisely this combination of linguistic fluency and underlying unpredictability that makes them problematic in situations where verifiable, systematic behaviour is paramount.”
LLMs are a lot of fun. But they can’t be trusted with anything important.
2. Why are LLMs and AI relevant to speech pathology?
As with almost all professions, many speech pathologists – including me – are experimenting with LLMs, including for research and clinical purposes. But, more importantly:
- some researchers think we need a completely different approach to create the next generation of AI language tools; and
- much to my excitement, they are looking at how humans acquire language to do it.
Here are some of the amazing things they are learning:
(a) Language is flexible
Language is incredibly complex and useful. For example, today I’ve used language to:
- ask a colleague questions about a client’s progress;
- request a much-needed long black coffee at my local cafe;
- schedule a zoom call;
- meet with clients;
- express my thoughts on the ‘spirit of cricket’ with an Englishman;
- exchange my beliefs on motor speech disorders and non-speech-related oral motor exercises;
- outline my opinion on the current NDIS pricing guidelines;
- describe my son’s school camp immersion;
- approve a management decision;
- take notes on a training webinar about supported decision-making;
- finish writing a resource featuring polysyllabic words containing split digraphs;
- explain to a friend how to get to the meeting point for my running group tonight;
- imagine what it will feel like to go on a future holiday at the end of next term;
- evaluate wardrobe renovation options;
- formulate and outline our plans for hiring additional clinicians;
- analyse what my wife might want for dinner tomorrow night, and predict what she will do if I suggest something else;
- build and pass on my knowledge of science fiction classics to my son;
- give instructions to my bank;
- complain about the Sydney Metro construction project;
- exchange quips with a friend;
- supervise a clinician;
- ignore several spammed emails;
- read updates on a quality improvement project; and
- write this blog.
And it’s only 4pm!
(b) Thinking and language are related. But they are not the same thing:
- Thinking is about goal-directed world-modelling, inference, and decision-making. We make models of the world based on our experiences and knowledge that can be updated with new information. These models support our predictions and decision-making toward our goals (e.g., Lake et al., 2017).
- Language is one way of communicating thoughts to others and receiving their thoughts in turn. It’s about mapping thoughts to external systems of symbols that represent our thoughts (e.g. speech sounds, spoken words, signs, glyphs, and written words), using these symbols to communicate thoughts to others, and translating others’ use of symbols to understand their thoughts (e.g. Lewis, 1978).
(c) Distinct brain systems and networks
Thought and language appear to operate in distinct (but interacting) brain systems. Neuroimaging studies reveal an anatomically separate “language network” in the frontal and temporal lobes of the brain specialised for processing language, which is closely connected to brain networks supporting other aspects of cognition (e.g. Mahowald et al., 2023), including problem-solving and reasoning. This “language network” is:
- activated in both language comprehension (e.g., Deniz et al., 2019) and language production (e.g., Hu et al., 2021);
- sensitive to regularities in all levels of language structure, from phonology, to words, to sentences (e.g. Blank & Fedorenko, 2017; Silbert et al., 2014; Molnar-Szakacs & Iacoboni, 2008); and
- implicated in combinatorial semantic and syntactic processing (e.g., Fedorenko et al., 2020 and Hu et al,. 2021).
By contrast:
- functional neuroimaging studies show the language network is not activated in a variety of non-language-related tasks, including reasoning about arithmetic, logic, actions, or events (e.g. Almatic & Dehaene, 2019; Paunov et al., 2022, and Shain et al., 2022); and
- networks other than the language network are activated in processing core cognitive domains, including logic, mathematical reasoning (e.g. Almaric & Dehaene, 2016)), social reasoning and planning (e.g. Adolphs, 2009), and physical reasoning and simulation (e.g. Pramod et al., 2022).
Some evidence suggests the existence of an “amodal semantic network” that:
- is physically close to the language network;
- may act as an interface between the language network and more general networks associated in non-language-related thinking; and
- may be activated in processing semantically meaningful sentences (e.g. Ivanova, 2022).
(d) Thought does not require language (Mahowald et al., 2023)
- Non-human species and preverbal infants are capable of modelling the world towards their inferences and goals without language (Spelke, 2022).
- Infants can model the world and draw inferences well before they know language (e.g. Gopnik, 1996).
- People with aphasia who have had localised damage to their language network (e.g. because of a stroke) can exhibit impaired language production and comprehension, but retain the ability to solve arithmetic and logic puzzles, reason about cause-and-effect and social situations, and perform other tasks that do not require language (e.g. Basso & Varley, 2007).
(e) Thinking comes first
- It seems obvious, but animals with brains think. Birds, bees, dolphins, crows and non-human primates, for example, all appear to be able to interpret the “noise” they receive from the world around them into cause-effect-based, explanatory world models that allow them to maintain coherent beliefs about the world and to infer consistent, useful predictions and plans. This allows them to solve useful problems based on their world models, including navigation, foraging for food, and physical predictions.
- Human intelligence stands out for its additional social reasoning abilities and our flexibility and expressiveness. Like non-human primates, dolphins and crows, we are creative: we can invent our own problems, as well as new approaches to solving them (e.g. Tomasello, 2022; Chu & Schulz, 2023).
- Unique to humans, we think about and understand problems far beyond what is necessary for our immediate survival, and consider goals and questions that require abstract, culturally-constructed and even hypothetical systems for modelling and understanding the world (e.g. Dennett, 2017).
- Infants are born equipped with a toolbox of thinking skills, including:
- an understanding of physical objects and events, and the goals and actions of others (e.g. Spelke, 2022); and
- general abilities for learning statistics and structure (e.g. Xu et al., 2021).
- Humans may compose and execute mental programs in an internal “language of thought” (Fodor, 1975), which is a structured symbolic system for representing conceptual knowledge that interacts with systems for reasoning and problem-solving.
(f) Human language emerges from scarce input
- As the name suggests, most Large Language Models are trained on vast data sets of human language; orders of magnitude greater than any human will encounter in a lifetime. LLM designers seek to extract representations of meaning from statistical patterns of how words are used in context (Wong et al., 2023). Some proponents of LLMs think that a sufficiently large data set is all that’s needed to create general intelligence (e.g. see Branwen, 2022), although most of the field seems sceptical about it.
- In contrast, most children acquire language skills from exposure to relative tiny amounts of language (e.g. Brown, 1973). Congenitally deaf children with no oral language input develop language to communicate their thoughts with the same basic hallmarks of natural languages like spoken English (e.g. Goldin-Meadow, 2012). From relatively sparse data, young children rapidly go beyond the utterances they hear to produce and understand entirely new utterances (e.g. Pinker, 1998). For more detail, read this article.
- Children then use language to acquire new concepts they would not get merely from direct experience (e.g. Carey, 2009).
- Language can be seen as a system of goal-directed actions for externalising and communicating thoughts to other intelligent beings (e.g. Goodman & Frank, 2016).
- For humans, language plays a profound role in determining the problems we think about, and how we think about them. We can communicate a broad range of our thoughts, including abstract and general world knowledge, our beliefs, questions, and our approaches to solving problems (Wong et al., 2023).
3. Bottom line: implications for future AI (and for more research about language)
Wong and colleagues think we can learn a lot that is relevant to the next generation of AI design by studying the relationships between human thought and language. Specifically, they think that future AI systems should be designed – not around language – but around thought:
“[G]eneral purpose computing systems that provide a principled framework for expressing world models, conditioning them on observations from sources including language and perceptual input, and drawing principled inferences and decisions with respect to the goals of an intelligent system”. (emphasis added)
Right now, of course, we’re a long way away from any kind of general artificial intelligence. But here’s hoping that one of the happy side effects of this interest will be significant new investment in research about human language acquisition, and language disorders.
Main source: Wong, L., Grand, G., Lew, A.K. Goodman, N.D., Mansinghka, V.K., Andreas, J. & Tenenbaum, J.B. (2023). From Word Models to World Models: Translating from Natural Language to the Probabilistic Language of Thought. Computer Science, preprint: arXiv:2306.12672.
This article also appears in a recent issue of Banter Booster, our weekly round up of the best speech pathology ideas and practice tips for busy speech pathologists, providers, speech pathology students, teachers and other interested readers.
Sign up to receive Banter Booster in your inbox each week:
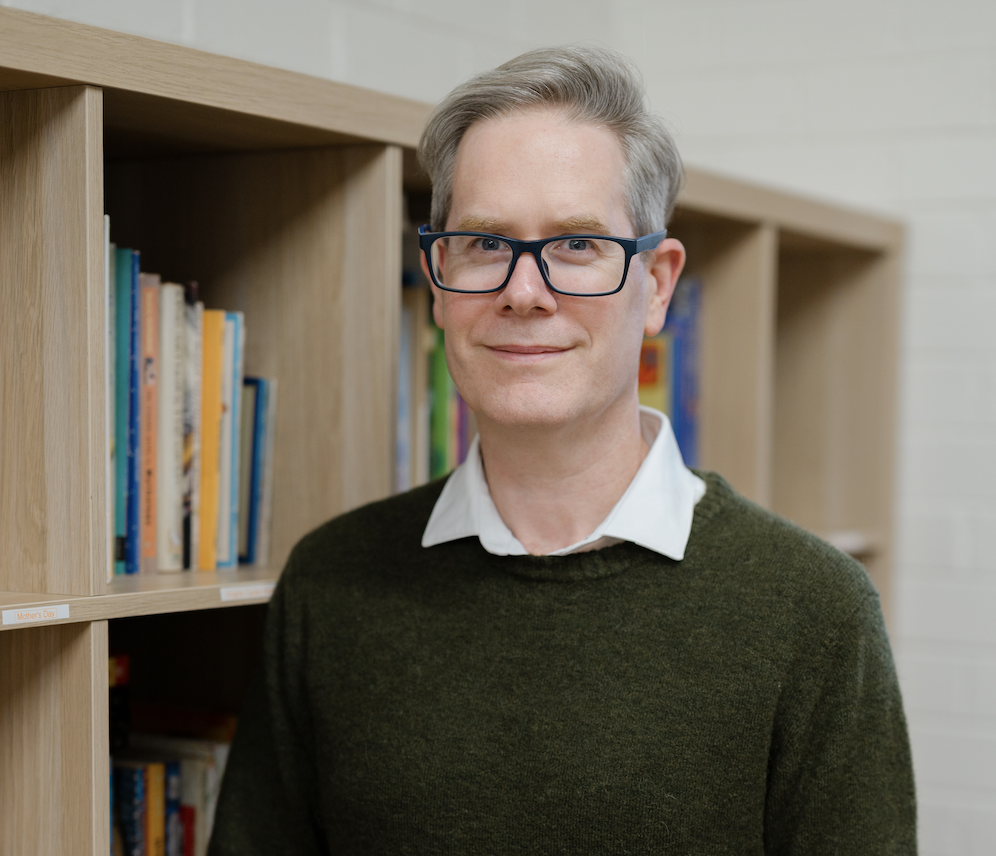
Hi there, I’m David Kinnane.
Principal Speech Pathologist, Banter Speech & Language
Our talented team of certified practising speech pathologists provide unhurried, personalised and evidence-based speech pathology care to children and adults in the Inner West of Sydney and beyond, both in our clinic and via telehealth.