Speech-language pathologists should pay close attention to research about large language models (LLMs)
With thanks to Mahowald, Ivanova, Blank, Kanwisher, Tenenbaum, and Fedorenko (2024), here’s why I think speech-language pathologists should pay close attention to research about large language models, like ChatGPT:
- You can learn a lot about dolphins by comparing them with sharks. You can learn a lot about human language by comparing it with large language models (LLMs), like ChatGPT.
- People routinely overestimate and underestimate LLMs:
- LLMs are remarkably good with formal language tasks requiring skills like: (i) phonology, (ii) morphology, and (iii) syntax.
- To date, LLMs are not so good with functional language tasks requiring skills like: (i) formal reasoning and problem-solving; (ii) world knowledge about people, objects, events, and ideas; (iii) dynamically tracking people, objects, agents, and events as narratives and conversations unfold; and (iv) understanding language in social contexts. LLMs do not perform well on some mathematical tasks, and regularly generate false statements called “hallucinations”.
- Humans have a language network in the frontal and temporal lobes of our brains (usually in the left hemisphere) that supports both comprehension and production of spoken, written and signed language, including on tasks requiring formal language skills. However, our brains also include other networks that support our use of language:
- formal reasoning tasks, like problem-solving, engage areas of the brain known as the multiple demand network, which is distinct from the language network but is involved even on verbal problem-solving tasks;
- language and semantic (real world) knowledge can be disentangled, as shown by case studies of people with aphasia (a language disorder caused by damage to the language network) and semantic dementia (a neurodegenerative disorder that can affect world knowledge);
- we can follow and recall conversations and stories by using language inputs to create mental (or situation) models using the default network, which tracks both linguistic and non-linguistic narratives; and
- our theory of mind network helps us to process social information, e.g. to infer other people’s mental states and intentions (with or without the use of language), and on non-literal language comprehension tasks, e.g. understanding jokes, sarcasm, and indirect requests.
- Some researchers think that LLMs will acquire more advanced functional language skills with enough language training. After all, many human non-language skills are enhanced with language inputs, e.g., when learning conceptual categories and facts about our world as children.
- Other researchers think that the next generation of LLMs should be trained to use language in more human-like ways. This may require the development or emergence of separate (but connected) modules for formal and functional language skills. This research may give us a better understanding of how human language works so we can better help people with formal and/or functional language challenges.
Source: Mahowald, Ivanova, Blank, Kanwisher, Tenenbaum & Fedorenko (2024). Dissociating language and thought in large language models. Trends in Cognitive Sciences (in press). Abstract: https://doi.org/10.1016/j.tics.2024.01.011
Related articles:
- ChatGPT is overrated because language and thinking are not the same thing
- What should I learn to write when I can just use ChatGPT and Grammarly?
- Parents: teach categories to your kids to ignite language development
- How do babies and toddlers learn language? (Spoiler alert: it’s incredible!)
- 68 FREE resources and tips to help your child improve their social skills by understanding what others are thinking and feeling
- Oral language: what is it and why does it matter so much for school, work, and life success?
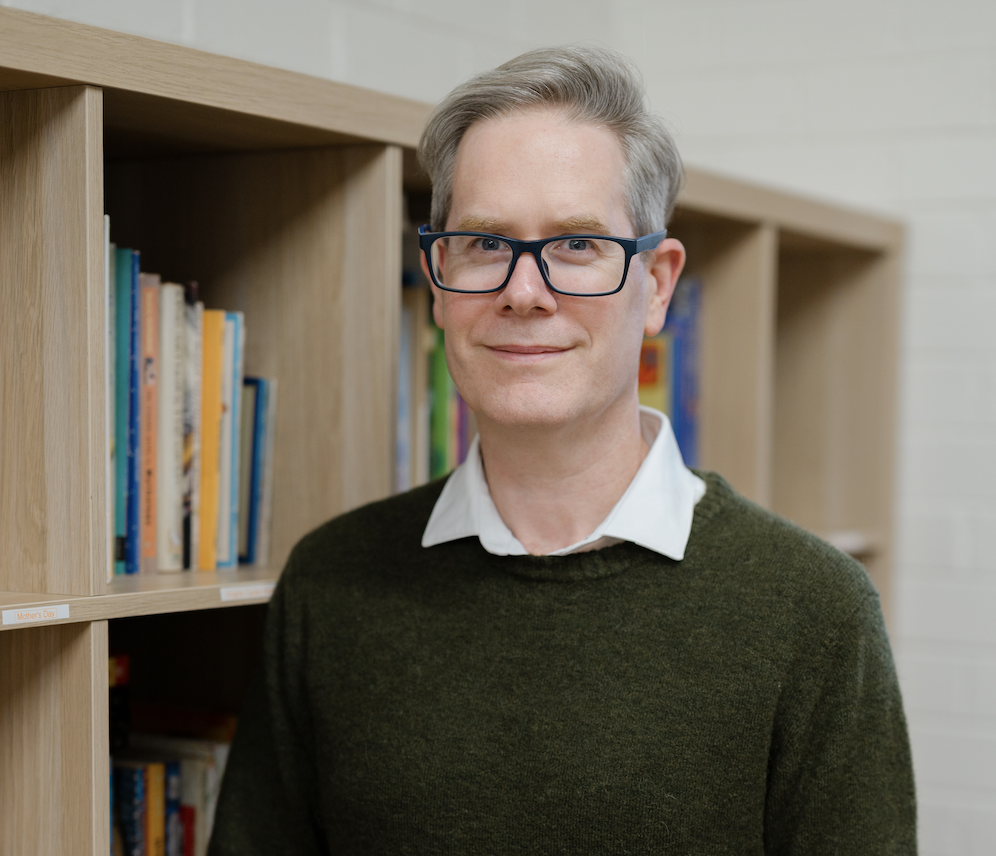
Hi there, I’m David Kinnane.
Principal Speech Pathologist, Banter Speech & Language
Our talented team of certified practising speech pathologists provide unhurried, personalised and evidence-based speech pathology care to children and adults in the Inner West of Sydney and beyond, both in our clinic and via telehealth.